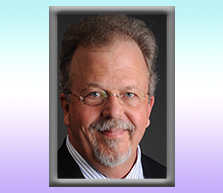
By Blackford Middleton, MD, MPH, MSc
Chief Informatics and Innovation Officer
Apervita, Inc
Introduction
Modern healthcare delivery is in transition. From the era of autonomous, provider-centric, discontinuous healthcare delivery, we are moving toward a more value-based and coordinated system of care delivery, and hopefully, a learning health system achieving the Quadruple Aim.1-3 To achieve this, however, we also need to move toward the next evolutionary stage in supporting health technology infrastructure to enable and sustain this transformation.4,5 Health IT in hospitals and health systems has evolved from departmental and mainframe hospital information systems to n-tier client-server architectures. We now need to deploy the inevitable next-generation architecture -- an industry-scale platform that:
This has been the progression in other industries.6 Digital health platforms (DHP) are emerging now in the post-EMR world to fully realize the value potential anticipated with the recent adoption of EHR.7
A DHP employs a cloud architecture to rapidly connect the dots in healthcare – across hospitals, clinics, 3rd-party consumers of healthcare data (accrediting bodies, quality assessors, and professional registries), payors, life-sciences, research, and even patients. It delivers the essential two-sided platform capabilities -- and platform economics -- to bring data producers and consumers together efficiently with dramatically reduced costs, improved data quality, and semantic data integrity from producer to consumer, thereby eliminating the need for intermediary services (and companies) that add complexity and expense to information exchange. The value proposition for an industry-scale platform in healthcare is clear – simply improving healthcare information exchange and interoperability is estimated to be worth $78B/yr,8 and that is only the tip of the iceberg. Additional savings arise from reduced medical error, closing quality gaps in care with clinical decision support, and reduced provider burden with administrative tasks such as prior authorization and more.9 As the data begins to flow through improved connectivity and interoperability, the value proposition only increases as the marginal cost of data acquisition goes down, and the marginal value of data goes up. This fundamental theorem of platform economics has been witnessed repeatedly in other industries – it is time for healthcare to experience the same benefit.
Transitioning Healthcare
To move toward value-based care, healthcare must improve its ability to define and measure the value of healthcare services and health itself. Advancing measurement science is improving our ability to assess the quality and efficiency of care, but current methods of quality measurement and reporting place far too great a burden on providers and health systems.10 This burden can be dramatically decreased with use of a digital health platform as the single infomediary between those reporting data for accreditation, quality assessment, or payment transactions, and those who perform those services. In fact, many of the same data may be re-used for different purposes on a digital health platform once acquired, underscoring the fundamental platform economics described above. As the ease of data acquisition for measurement increases and the burden decreases, health care organizations will have the opportunity to more fully measure health care processes, cost, and quality without burdening the provider. They will also be able to more fully ‘instrument the healthcare enterprise’ with a wide variety of assessments and measures that will fully enable a data-driven approach to defining and managing a healthcare production function in real terms. Payors, researchers, and public health authorities will have an improved ability to conduct ‘systemic’ assessments of the efficiency of healthcare delivery -- whether it be:
This improved information exchange and measurement will also enable possibly the most dramatic transformation of the care delivery ecosystem – beyond interoperability of healthcare data, the DHP enables true ‘interoperation’ of health care enterprises on behalf of the patient. Interoperation is a new term – meaning the ability of healthcare enterprises to not only efficiently exchange health data, and measure care delivery, but also the ability to truly collaborate in care delivery and assessment, and even public health. Initially this will be enabled by improving the connectivity between payors and healthcare delivery systems for the purposes of prior authorization, e-payment, patient referral, etc.11 Ultimately, it will also enable caring for patients in a truly collaborative fashion among providers where patient data and their care plan follows the patient from one point of care to the next. Previously separated worlds of health information management across clinical care, public health, research, life-sciences, and the consumer will converge at least in part. This will lead to the ability to not only repurpose data in a secure and confidential manner, but also the ability to share knowledge and deliver insights across the care continuum from one information world to another. For example: delivering care management guidance for an STD (sexually transmitted disease) to the point of care and using the same underlying logic to do case reporting to the public health authorities as appropriate.
Transforming Healthcare
Such knowledge sharing will enable what undoubtedly will be the ultimate transformation of care toward a truly learning health care system. This will occur when the best available evidence for each and every clinical decision is available to decision-makers: patients, or patient-provider pairs, to enable ‘best care everywhere’.12-14 And, when best evidence from clinical guidelines is not available, we can use machine learning and artificial intelligence to learn from the aggregated data at the patient level (precision medicine), population level, or community and public health level of analysis.15 We can alleviate the challenges of seeing a patient without the complete record being available, the best evidence relevant to the decision at hand being available, or the key social and community determinants of health being known. Ultimately, low-level task and process automation can facilitate the clinical workflow through secure exchange of documents and data, leaving higher level decision-making functions to the clinician and the care team. Thus, the patient can be supported by advanced cognitive aides to decrease delayed or missed diagnoses, medical errors, or quality gaps in care, and fundamentally optimize care in ways previously unimaginable.16,17 With appropriate built-in feedback and performance monitoring from the instrumentation above, we will also enable at last a learning health system.
These are exciting and turbulent times in healthcare today. A digital health platform is not the only answer, but it is a critical prescription to help cure what ails healthcare.
References
- Berwick DM. Era 3 for medicine and health care. JAMA. 2016;315(13):1329-1330.
- Friedman CP, Wong AK, Blumenthal D. Achieving a nationwide learning health system. Science translational medicine. 2010;2(57):1-3.
- Sikka R, Morath JM, Leape L. The quadruple aim: care, health, cost and meaning in work. BMJ Qual Saf. 2015;24(10):608-610.
- Blumenthal D, Glaser JP. Information technology comes to medicine. New England Journal of Medicine. 2007;356(24):2527-2534.
- Blumenthal D. Launching HITECH. New England Journal of Medicine. 2010;362(5):382-385.
- Bush J, Fox J. Bringing the Power of Platforms to Health Care. Harvard Business Review. 2016.
- Middleton B. Chairman’s column: health informatics and healthcare transformation-entering the post-EMR era. Journal of the American Medical Informatics Association. 2014;21(6):1141-1142.
- Walker J, Pan E, Johnston D, et al. The value of health care information exchange and interoperability. Health Affairs. 2005;Suppl Web Exclusives:W5-10-W15-18.
- Middleton B, Cheung NT. Health Information Technology and Value. In: Sheikh A, Cresswell K, Wright A, Bates DW, eds. Key Advances in Clinical Informatics: Transforming Health Care through Health Information Technology. London, UK: Academic Press; 2017.
- Holman GT, Waldren SE, Beasley JW, et al. Meaningful use's benefits and burdens for US family physicians. J Am Med Inform Assoc. 2018.
- See HL7 Da Vinci Project.
- Dixon BE, Simonaitis L, Goldberg HS, et al. A pilot study of distributed knowledge management and clinical decision support in the cloud. Artificial intelligence in medicine. 2013;59(1):45-53.
- Middleton B. The clinical decision support consortium. Studies in health technology and informatics. 2009;150:26-30.
- Kawamoto K, Hongsermeier T, Wright A,et al. Key principles for a national clinical decision support knowledge sharing framework: synthesis of insights from leading subject matter experts. Journal of the American Medical Informatics Association: JAMIA. 2013;20(1):199-207.
- Jordan MI, Mitchell TM. Machine learning: trends, perspectives, and prospects. Science. 2015;349(6245):255-260.
- Patel VL, Shortliffe EH, Stefanelli M, et al. The coming of age of artificial intelligence in medicine. Artificial intelligence in medicine. 2009;46(1):5-17.
- Middleton B, Sittig DF, Wright A. Clinical decision support: a 25 year retrospective and a 25 year vision. Yearb Med Inform. 2016;Suppl 1:S103-116.
About the author:
Blackford Middleton is Chief Informatics & Innovation Officer at Apervita, Inc., a provider of a digital health platform to democratize healthcare data and analytics. Previously, he was a Professor of Biomedical Informatics and Medicine, at Stanford, Harvard, and Vanderbilt Universities, and he held executive leadership roles at Stanford (CMIO), MedicaLogic/ Medscape (CMO), Partners Healthcare System (Director Clinical Informatics R&D), and at Vanderbilt (CIO). Dr. Middleton’s work is focused on clinical informatics – the applied science surrounding strategy, design, implementation, and evaluation of clinical information systems in complex environments.
Middleton was appointed by US Secretary of Health and Human Services Michael Leavitt to serve on the National Committee of Vital and Health Statistics (NCHVS) and served from 2008-12 on the Quality Committee (Co-Chair), and Population NCVHS sub-committees. He is past Treasurer of the American Telemedicine Association, and of the American College of Medical Informatics, and past Chairman of the Computer-based Patient Record Institute (CPRI, 2000), and past Chairman of the Healthcare Information Management & Systems Society (HIMSS, 2005). Previously, Dr. Middleton was a member of the Board of Directors at the American Medical Informatics Association (AMIA) and was Chairman of the Board (2014-15). He served on the Board of Stewardship Trustees for the Catholic Health Initiatives (CHI) health system (and Quality, and Strategy sub-committees of the Board) from 2006-12, and the Board of Directors of the CHI Institute for Research and Innovation from 2009-12.
Dr. Middleton studied Biochemistry and Molecular Biology at the University of Colorado, Boulder. He received a Master’s degree in Public Health from the Yale University School of Public Health, with a dual concentration in Chronic Disease Epidemiology and Health Services Administration. He received an MD from SUNY Buffalo, and was a resident in internal medicine at the University of Connecticut Health Sciences Center. He completed an AHCPR Fellowship in General Internal Medicine at Stanford University, where he received his Master of Science degree in Health Services Research, focusing on clinical informatics.