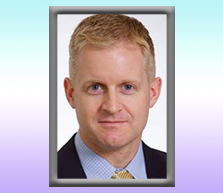
By Davis Walp, MBA
Founder / CEO Health Analytics Strategies LLC
Healthcare struggles with a definitional problem – where and how to invest scarce resources in order to drive health value. In 2007, the IOM Roundtable on Evidence Based Medicine published the first in a series focusing on the "learning health systems" that raised issues important to improving the development and application of evidence in healthcare decision-making. While many of these principles have been broadly adopted, we do a poor job, in the US, as evidenced by the rate of growth in healthcare spending relative to growth of GDP and resulting health outcomes that underperform our nation-state’s peers across a range of therapeutic areas. The purpose of this brief commentary is not to try to diagnose or solve how we optimize healthcare system performance, but to (1) frame a conversation around the sufficiency of contemporary data and health analytics in helping researchers characterize cause-and-effect relationships in healthcare, and (2) highlight critical questions surrounding the application of new sources of patient data and research analytics that will be powered by an emerging digital health ecosystem. There is great promise in digital health data to help us better understand and drive human health, but there are also significant hurdles that need to be addressed before we can realize the potential.
There is little debate that digital health is an area of high focus and investment across the healthcare ecosystem. According to Rock Health, in 2018, $8.1B of venture capital were invested in digital health technology, up 42% from 2017.2 These are technologies (such as mobile applications, personal devices, and wearable sensors) that can empower consumers to make better-informed decisions about their own health and provide new options for facilitating prevention, early diagnosis of life-threatening diseases, and management of chronic conditions outside of traditional care settings. The FDA expands this definition to include software and technologies that assist in diagnosis, treatment options, storing and sharing health records, and managing workflows to enable more efficient clinical practice.3
While there is almost universal consensus around the potential of digital health to move the needle, I consider it a nascent healthcare segment composed of a fragmented collection of industry participants. I expect that we will observe significant evolution in the space and steadily increasing impact on human health. I argue that it is imperative to build “learning health system” principles into the foundation of the digital health ecosystem. This presents a “call for action” to the research community to lead in shaping the standards, the methods, and defining best practices around the capture of longitudinal patient-level data that will transform health services research and ultimately the practice of medicine.
Across the healthcare ecosystem today, providers, payers, biopharmaceutical companies, diagnostic device manufacturers, policy makers, and health technology players are all focused on surrounding providers with the tools that they require to appropriately diagnose and optimally treat each patient along each phase of that patient’s journey. For a moment, let’s put aside the complex debates around interoperability, payment models, risk sharing, care process transformation, health policy, etc. The core of value in health is measured at the individual patient level. Researchers have very complex measures for describing clinical, economic, and humanistic value and the healthcare ecosystem is relying on these measures of value to determine how to improve payment models and care processes, and arm providers with the tools that will drive health value for their patients.
In my opinion, much of the failure to improve health system performance is due to insufficiency around generating insights and evidence. I argue that health researchers are very proficient at asking good questions and selecting the right methods to make sense of data, however the data we either observe or collect through rigorously-structured studies is generally incapable of representing all of the factors that explain variance in outcomes of interest. This manifests in the form of high levels of heterogeneity in the outcomes of interest, as a result of many factors, including:
There is an analogy from the high-end audio world that fits well. While many of us enjoy digital audio, some audio enthusiasts will argue that the digital representation of the music is inferior to the original analog waveform that was originally created during the performance. For example, the Red Book CD Standard1 is based off of sampling the original analog waveform 44,100 times per second and describing each sample with a 16-bit “word.” Audio enthusiasts will argue that we are throwing away the majority of the information in the original waveform that falls between each sample.
There are direct corollaries in healthcare analytics. In the real-world evidence analytics space, the data coming from the usual care setting is notoriously incomplete and error-prone. Clinical trialists and late phase researchers routinely structure siloed protocols in order to optimize for time, complexity, and available budget. As a result, researchers are highly accustomed to seeing differences in the effect of new interventions in the real world compared to what was estimated in clinical studies or modeled by health plans or quality improvement teams. A large part of this has to do with high levels of variability that are the result of “incomplete sampling strategies.” This is the reason that digital health holds great promise from a research standpoint to “fill in the gaps” and create denser data sets with greater explanatory power.
We need to prepare for a world where we can capture streaming biometric data from wearable devices or apps, correlate consumer behaviors with progression of disease and treatment response, and correlate the emotional and cognitive states of patients with their healthcare journey. This implies that we need new ways to think about how to capture, transform, semantically harmonize with other health data, apply methods, and appropriately respond to the findings. We also need new decision support paradigms at the point of care that will enhance clinical decision-making. There are companies who have begun to tackle this (eg, Evidation captures, quantifies, and analyzes health behavior data).4
The application of research analytics to this data is an important topic that is gaining traction among the research community. In the presence of validated measures, there is a clear pathway to application of statistical models that will estimate overall trends, as well as isolate the effect of specific variables.5 There is a need, however, to identify where and how researchers and analysts can deploy machine learning and artificial intelligence to support valuable use cases such as signal detection, insight generation, or hypothesis development / validation. ISPOR has recently formed a special interest group focused on Digital Health with the remit to educate and engage ISPOR members within the topic area to advance health economic and outcomes research and the use of this research in health care decisions.
I have little doubt that digital health will drive meaningful impact in human health. There is significant opportunity to advance health services research, but this will require strong and vocal representation from the research community, with focus and continued investment on data standards, supporting technology, and methods. The insights and evidence we generate will have the opportunity to re-align our fundamental understanding of human health and shape our strategies for driving health value at the individual and population level. This is an exciting future that I am personally excited to participate in.
References:
- https://en.wikipedia.org/wiki/Compact_Disc_Digital_Audio
- https://rockhealth.com/reports/2018-year-end-funding-report-is-digital-health-in-a-bubble/
- FDA - Digital Health Innovation Action Plan
- https://evidation.com/research https://www.fharrell.com/post/stat-ml/
- https://www.fharrell.com/post/stat-ml/
About the Author:
Davis Walp is the Founder / CEO of Health Analytics Strategies LLC, a provider of health data brokerage and advisory services to small and large biopharma, medtech, health plans, and investors. He has 23 years of experience across lifesciences manufacturer, professional services, and health information technology segments and has a track record of corporate strategy, partnership development, and general management of several healthtech startups spanning precision medicine, RWE data analytics, clinical and observational research, and patient engagement.